Kaspersky Lab WorkSpace Security EU ED, 10-14u, 3Y, RNW KL4851XAKTR ユーザーズマニュアル
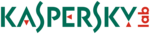
製品コード
KL4851XAKTR
U
S E R
G
U I D E
102
2. If a message was sent using Microsoft Exchange Server and scanning of such messages is disabled, the
message will be assigned the not spam status.
3. The message is subject to the analysis for lines from the list of allowed phrases. If at least one line from this list
has been found, the message will be assigned the not spam status.
4. The message is subject to the analysis for lines from the list of banned phrases. Detection of words from this list
in the message increases the chances of the messages being spam. When the calculated probability is over
100%, a message will be assigned the spam status.
100%, a message will be assigned the spam status.
5. If message text contains an address included into the database of suspicious and phishing web addresses, the
message receives the spam status.
6. The application analyzes the email message using the PDB technology. While doing it, Anti-Spam compares the
headers of mail messages with the samples of headers of spam messages. Each match increases the
probability that the message is in fact spam.
probability that the message is in fact spam.
7. The application analyzes the email message using the GSG technology. While doing it, Anti-Spam analyzes
images attached to the email message. If the analysis reveals signs typical of spam in the objects attached to
the message, the probability of it being spam increases.
the message, the probability of it being spam increases.
8. Anti-Spam analyzes email message using Recent Terms technology. While doing it, Anti-Spam searches for
phrases characteristic of spam in the text. These phrases are contained in the updatable Anti-Spam databases.
After the analysis is complete, Anti-Spam calculates how much the probability of the message being spam has
increased.
After the analysis is complete, Anti-Spam calculates how much the probability of the message being spam has
increased.
9. It checks for the presence of the additional features (see section "Using additional spam filtering features" on
), typical of spam. Each detected feature increases the probability that the message being scanned is
in fact spam.
10. If Anti-Spam has been trained, the message will be scanned using the iBayes technology. The self-training
iBayes algorithm calculates the probability that the message is spam, based on the frequency of occurrence of
phrases characteristic of spam in the text.
phrases characteristic of spam in the text.
The result of the message analysis is the probability that the email message is spam. Spam creators constantly improve
spam camouflage, therefore the calculated probability does not reach 100% in most cases. To ensure efficient filtering of
the email message stream, Anti-Spam uses two parameters:
spam camouflage, therefore the calculated probability does not reach 100% in most cases. To ensure efficient filtering of
the email message stream, Anti-Spam uses two parameters:
Spam rating
– probability value, which will cause the message to be considered spam when exceeded. If the
probability is below this threshold value, the message is assigned the potential spam status.
Potential spam rating
– probability value, which will cause the message to be considered potential spam when
exceeded. If the probability is less than this value, Anti-Spam will consider the message not spam.
Depending on the specified spam and potential spam ratings, messages will be assigned the spam or potential spam
status. Messages will be also assigned the [!! SPAM] or [!! Probable Spam] label in the Subject field. Then they are
processed according to the rules (see section "Actions on spam" on page
status. Messages will be also assigned the [!! SPAM] or [!! Probable Spam] label in the Subject field. Then they are
processed according to the rules (see section "Actions on spam" on page
) you have created for your mail client.
S
EE ALSO
Anti-Spam ......................................................................................................................................................................
T
RAINING
A
NTI
-S
PAM
One of the most powerful spam detection tools is the self-training iBayes algorithm. This algorithm makes a decision
about the message's status based on the phrases it includes. Before starting, sample strings of useful and spam mail
should be submitted to the iBayes algorithm to train it.
about the message's status based on the phrases it includes. Before starting, sample strings of useful and spam mail
should be submitted to the iBayes algorithm to train it.